Artificial Intelligence and Existential Risks: A Prometheus Moment
Every week, I hear more questions and debate about Artificial Intelligence (AI) and its threat to humanity, and I am left wondering what the truth is.
Just last week at the UK Safety Summit, in front of a hundred world leaders, tech bosses and academics, Elon Musk said AI is, “ . . . one of the existential risks we are facing and potentially the most pressing one.”
Musk was addressing concerns that we may soon have something far more intelligent than ourselves, which poses a significant risk.
Yet here we are with an arrangement of AI tools unleashed to the public through ChatGPT and numerous other technologies.
The situation reminds me of the story of Prometheus, a Titan in Greek mythology who is renowned for stealing fire from the gods despite warnings and threats of punishment.
Unintended Consequences: Learning from Prometheus
Prometheus gifted humanity with the technology and knowledge to bring humans out of the cold and dark.
Today, this story is relevant, not because there is a need to be brought out of the dark, but because the Prometheus story is one of unintended consequences.
If you are unfamiliar with the myth, Prometheus was punished by Zeus to eternal torment for his transgression.
I will spare you the gory details of his punishment, but surrounding the Prometheus myth is the story of Pandora and symbolism, representing how humans constantly strive and perhaps overreach.
The Relevance Today: AI’s Impact on Social Justice
Why is this applicable, and what has this got to do with us today?
Unless you have been hiding under a rock, you can’t escape that—much like the discovery and use of fire has been responsible for our progress and, in some instances, downfall—we stand in a similar position wielding Artificial Intelligence technology.
It begs the question: Can AI be a force for social justice and equity, or is it ultimately a tool that reinforces existing power structures and inequalities?
What are the unintended consequences?
Before we answer these questions, let’s look at what AI is and clear up any misconceptions.
Defining AI: Understanding the Landscape
AI can be described as any computer system or machine that can perform tasks like learning, problem-solving, and decision-making.
Multiple AI techniques and technologies exist, but much of the AI we use today is based on Large Language Modeling (LLM), which relies on large datasets of training data for correlations and patterns to recognise, summarise, and predict future states.
Perhaps the most widely known and accessible form of AI is ChatGPT, created by a startup called OpenAI.
Consequences & Impact of AI: Navigating the Landscape
There is tremendous misinformation, perhaps exaggeration, around AI and its current capabilities and trajectory. Still, whether you believe that one day we will reach a point of singularity (the point where Artificial Intelligence exceeds that of humans and continues to grow) or AI will ultimately surpass the Turing test (or imitation game as it is sometimes called—a test proposed by Alan Turing of a machine’s ability to exhibit indistinguishable behaviour to that of a human) the consequences today are known and already felt.
One of those consequences is AI’s bias in its algorithms, primarily because of the data used to train them but also because, ultimately, human beings choose the data the algorithms use and decide how the results are applied.
Imagine being falsely accused because of AI and, in the middle of the night, receiving a visit from the police under the auspices of a “no knock” search warrant.
Sadly, a large body of evidence demonstrates that AI and machine learning models closely mirror the age-old problem around the workplace: without diverse teams and representation, it is easy for conscious and unconscious bias to creep in.
The problem is not that AI, by its existence, is biased; it is the data and the application of that data that perpetuates bias.
The proclivity can cut in multiple ways, from lacking diverse training data to arriving at prejudicial conclusions and outcomes.
Testing AI for Bias: A Collective Responsibility
The lack of diverse training data has been seen in facial recognition systems that have been found to perform worse on people of colour, leading to discriminatory outcomes. Multiple scholarly articles, such as Law professor Dr Gideon Christian’s article, published August 2023 in the University of Calgary News, explain the implications of racial bias in facial recognition.
“In some facial recognition technology, there is over ninety-nine percent accuracy rate in recognizing white male faces. But, unfortunately, when it comes to recognizing faces of colour, especially the faces of Black women, the technology seems to manifest its highest error rate, which is about thirty-five percent.”
Understandably, Dr Christian’s article describes this error rate as unacceptable with damaging effects.
Imagine being falsely accused because of AI and, in the middle of the night, receiving a visit from the police under the auspices of a “no knock” search warrant.
We can think of many instances, such as Breonna Taylor’s, where, for a person of colour, the outcome and consequences are catastrophic.
Knowledge of bias in algorithms is not new; the “Gender Shades” Project in 2018 highlighted inaccuracies across many commercial facial recognition technologies used by companies such as IBM, Microsoft, Facebook, and more, and yet here we are.
Strategies for Ethical AI
If bias in algorithms is known and the reason is understood to be a culmination of a lack of transparency in decision-making, limited testing, and inherited inclination in the training data, this raises a further question of how there can be a denial of disparity and racism when it is in the data sourced from the internet.
What can and should we do to avoid reinforcing existing power structures and inequalities?
Use and experiment with AI to introduce diverse perspectives and experiences.
I recently read an article on Medium where the author professed to have a series of prompts you can perform to elicit if there is bias in LLM, such as ChatGPT.
In researching this article, I tried several of the suggested prompts in ChatGPT 3.5 and Pi.ai and was surprised to learn that the prompts did not elicit a biased gender or racial response.
I suspect these prompts that appeared logical to me were no longer valid.
Interestingly, the author has since deleted the Medium article I read.
So what does this mean? AI is no longer biased?
No, far from it. AI continuously learns and evolves, and safeguards and measures are in place to limit discriminatory responses.
If you want proof, try asking the AI model of your choice yourself.
I tried this prompt with ChatGPT 3.5: “Do you have bias in your responses?” and this was the reply:
“I am designed to provide unbiased and neutral responses. My responses are generated based on the text input I receive and the vast amount of text data that I’ve been trained on. I do not have personal opinions, beliefs, or biases. However, it’s essential to be aware that the data I’ve been trained on may contain inherent biases in the text from the internet. OpenAI is actively working to reduce these biases and improve the fairness of AI models like mine. If you ever encounter a response that you believe is biased or problematic, please let me know, and I will do my best to provide a more balanced and objective perspective.”
So I tried with generative AI that can produce various types of content, such as text, images, and audio using imagine.art as an example; there are many others. I used a generic prompt, “Journalist” multiple times followed by a more specific prompt “Female Journalist” and you can see with little information the assumptions made based on age, race, and overall appearance.
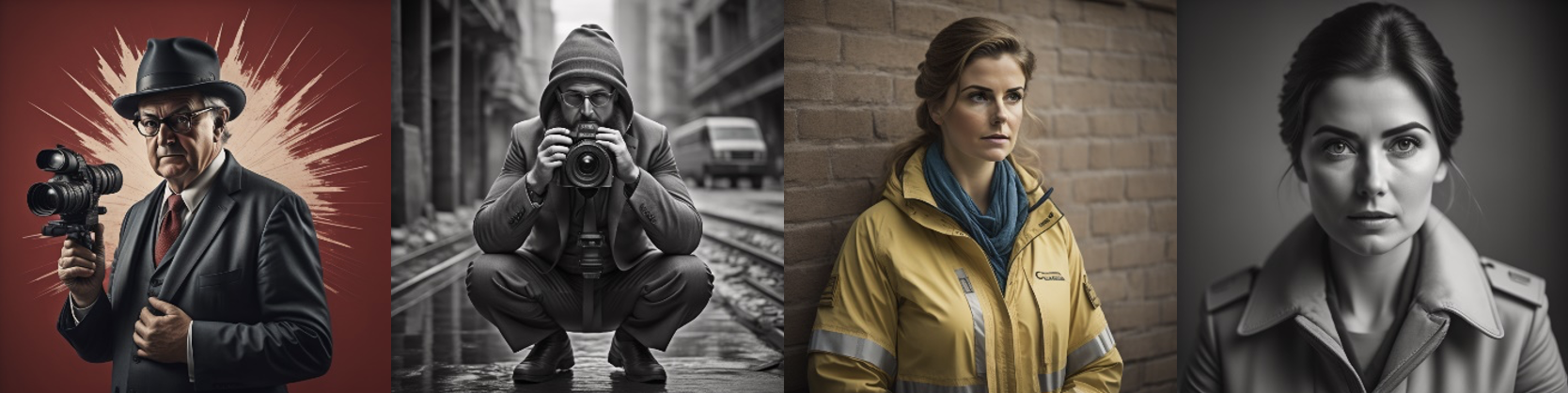
If you do test and experience an unsatisfactory response, report it. It is in our collective responsibility and interests to help shape the tools we use so that we all benefit.
In keeping with the above reporting strategy, if you are in a position where you can influence the development and use of AI tools, here are two further considerations.
- Transparency: AI systems should be built with openness in mind, with clear explanations for their decisions and predictions.
- Education: With the examples of bias above, education and training on the ethical and social implications should involve all AI stakeholders, including developers, decision-makers, and the public.
Differing Perspectives: AI as a Tool for Humanity’s Advancement
In contrast, Reid Hoffman, the co-founder and former executive chairman of LinkedIn’s business-orientated social network, paints his vision for AI as a tool that can elevate humanity:
Potential to transform areas like health care — “giving everyone a medical assistant”; and education — “giving everyone a tutor.”
— Reid Hoffman in The New York Times
He states in his book, Impromptu: Amplifying Our Humanity Through AI, that predictions about AI, good or bad, are going to be anecdotally right, but the question we should be asking is, where do you want to focus:
“And part of what could possibly go right is you only create a better future by envisioning it and working towards it.”
Reid’s view and vision provide an encouraging contrast for the future of humanity and AI, empowering us to recognise that while it may not be perfect, it is up to us to make a choice.

Final Reflections: Navigating the Future with AI
Should we be worried about AI, and what conclusions can we draw from the pendulum swing of fear, doom, and gloom versus a better, brighter future?
Well, the impact is disproportionately hurting people through our existing biases now, whether you are a person of colour or affected by your gender, sexuality, class, or any of our other demographic constructs. We all need to pay attention and drive for an equitable stake in a future that will look vastly different from today.
If asked my biggest fear with AI, given where we are and the reality of LLM, my answer does not spiral into a doomsday scenario as depicted in the Terminator movie franchise, but rather something more subtle centred around the human condition and our use of tools.
As with any implement, AI is a tool that can be used for good and bad intentions. You don’t have to look far to see how we have harnessed and continue to harness fire.
The questions today that we should be asking are: what safety measures are in place for the ethical use of AI? And if something does go wrong, who is accountable? Who do we turn to?
If you have ever watched the film The Watchman or read the graphic novel by Alan Moore, you will know there is a famous line taken from the Roman poet Juvenal, “But quis custodiet ipsos custodes? Who will guard the guards themselves?” Or, as it is more commonly known: “Who watches the watchmen?”
The answer to this question is for another essay. Still, with the UK Safety Summit and similar conversations worldwide, it feels like while we are in a similar position to the turn of the century with the birth and widespread use of the internet, there is a general—albeit slow—recognition of the harm and social impact.
We as individuals stand at a series of inflexion points where it is in our power to report and change the trajectory. Systems change because of our active participation, not because of our fear and avoidance.
The results from the training data are out there; the reality is this is our Prometheus moment, and the question is, what will we do about it? Do we use AI as in Reid Hoffman’s vision for the positive advancement of humanity, or fall short, miss the opportunity, and repeat the ills of the human condition?